DATA CENTERS 3.0: ‘MAN vs MACHINE CRYPTO-MANIFESTO’ !
IT has come to the knowledge of much-advised ADMPers that in the 21st century, giant data centers clusters will be faced with GINORMOUS challenges ranging from DATA Pipelines, to the right balance between edge computing, and Fog computing, or managing the A.I. ….
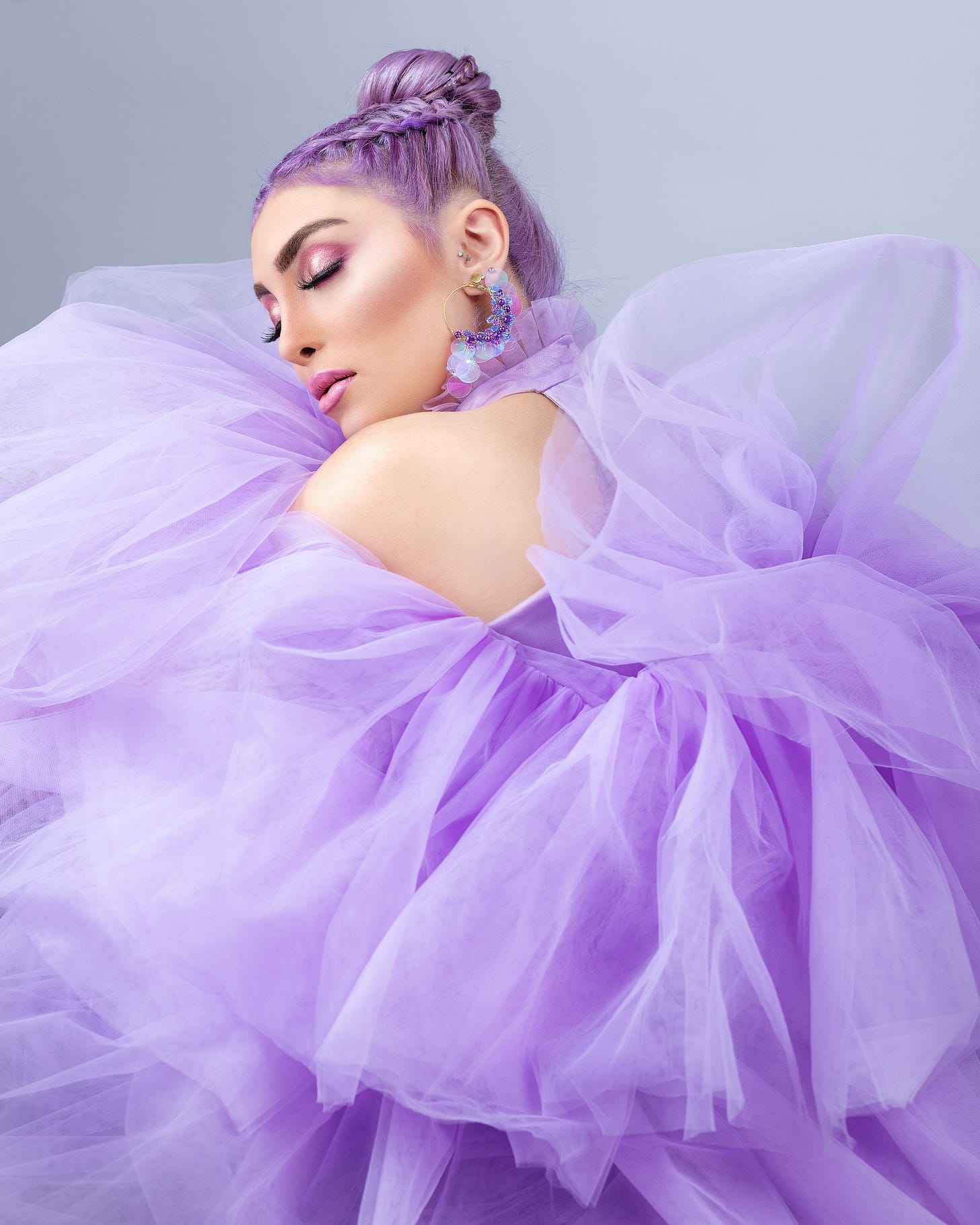
The second challenge, will come at staff level and the right mix of Data scientists Vs Data engineers Vs Data analysts Vs ‘On-the-ground observers’, and easing in the new jobs and tasks created by agile management-style procedures.
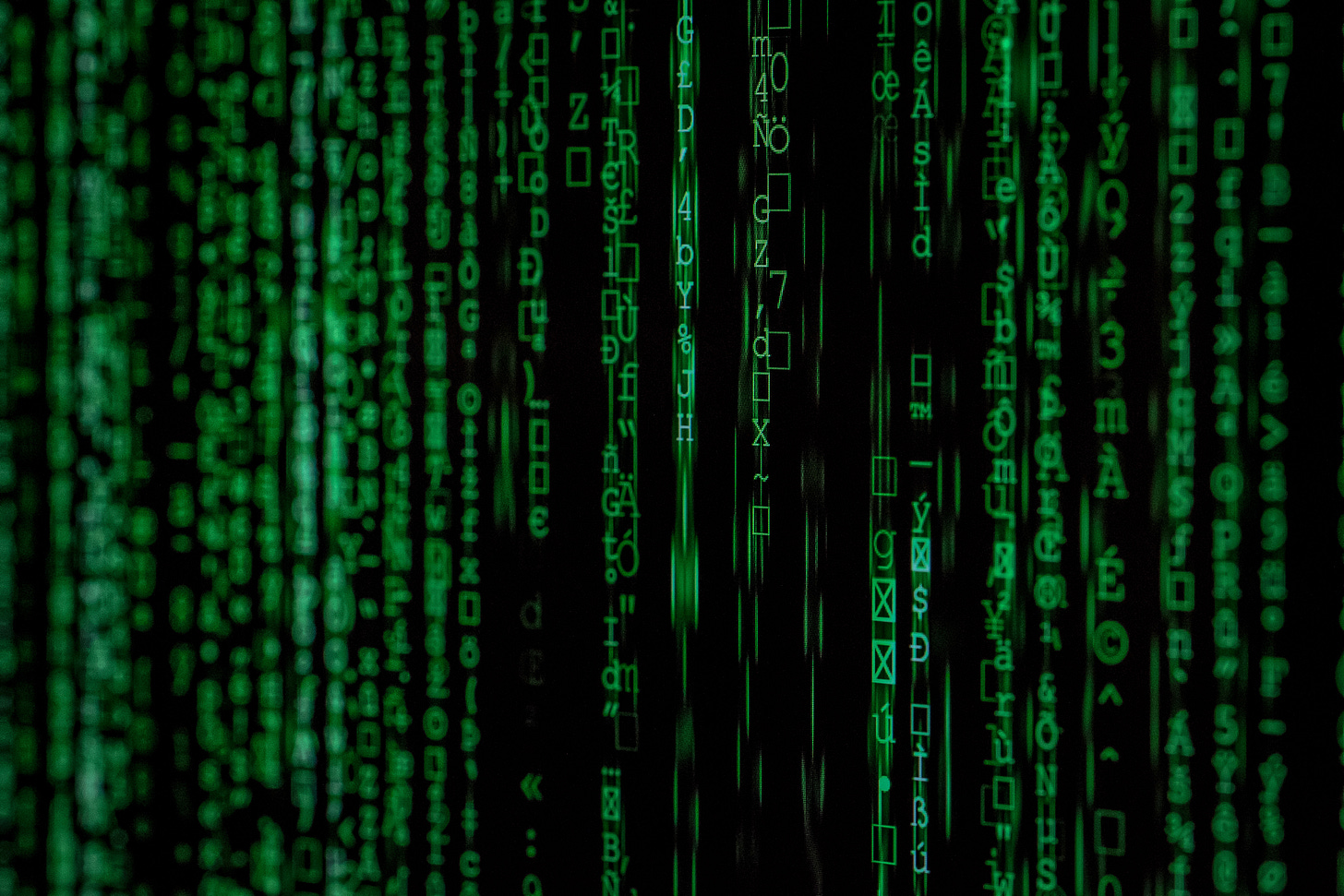
A./> TIME TO SECURE THE PROVERBIAL DATA “BAG” ?
ADMPers have now come up with a manifesto that should ease the process vastly, the same way the ‘Agile Manifesto’ was intended as a set of principles and guidelines rather than ‘Unbreakable red lines in the sand” …
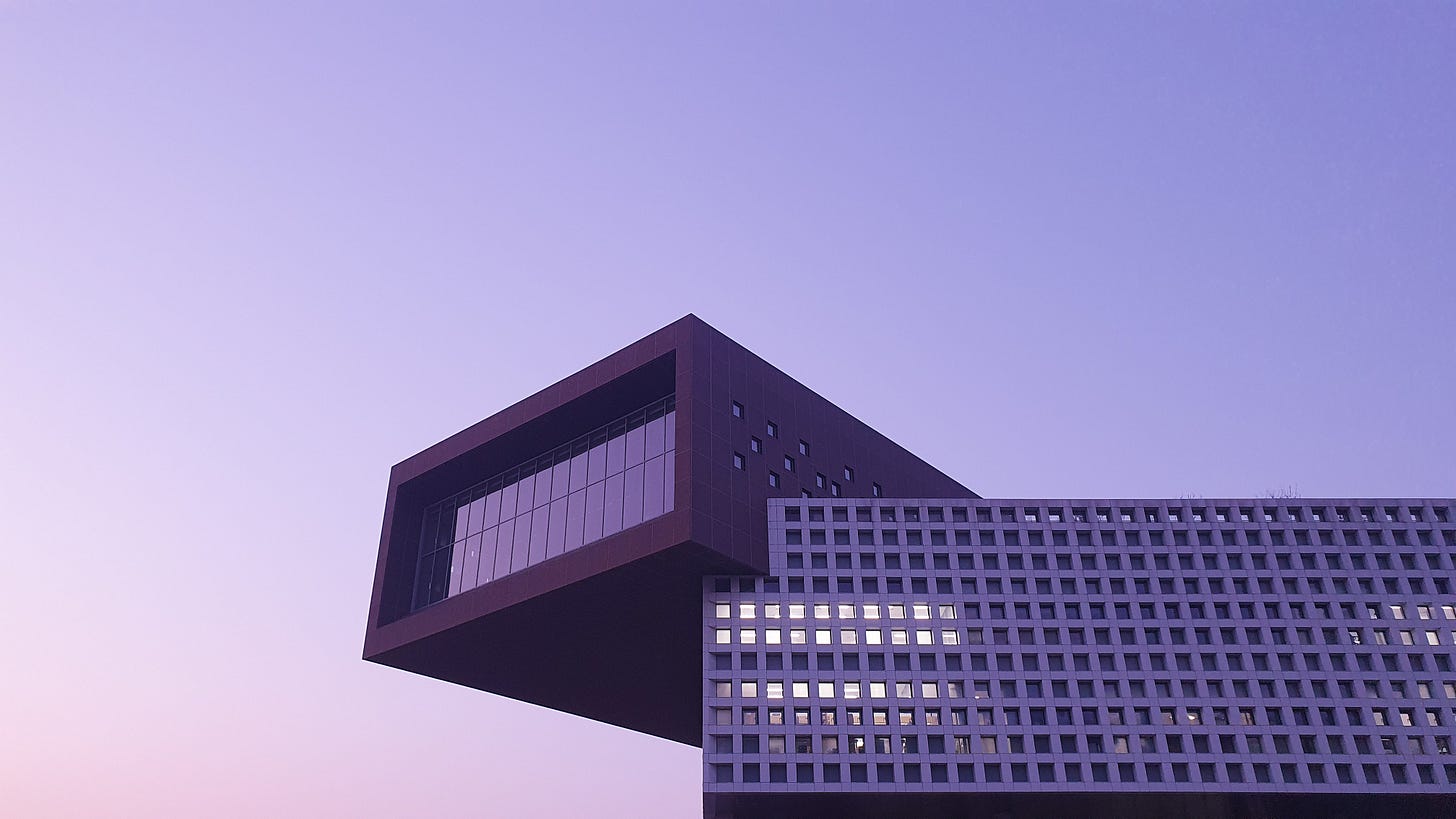
We operate not as “Boomers” of course, but rather, as …what you would call “Zoomers”:))=
Meaning: We do have a Blockchained Data-driven, and analytics-driven way of thinking and operating.
B./> MAN vs MACHINE in DATA CENTERS, MANIFESTO :
The collect, treatment, and management of BIG DATA and METADATA, must include a regular procedure of destruction, cleaning and re-classification (re-labelling) of all those datas.
Data is money, therefore money is Data. It stricktly correlates the ‘use of Data’, to an intrinsic and even monetizable value, that will evolve with time…
Human beings will create a digital footprint, from the day they are born, untill the day they die, and sometimes beyond, when it commes to connections, memory, arts, commerce, rememberance,… “Biological Human Data or, Organic Human Data” will have to be considered
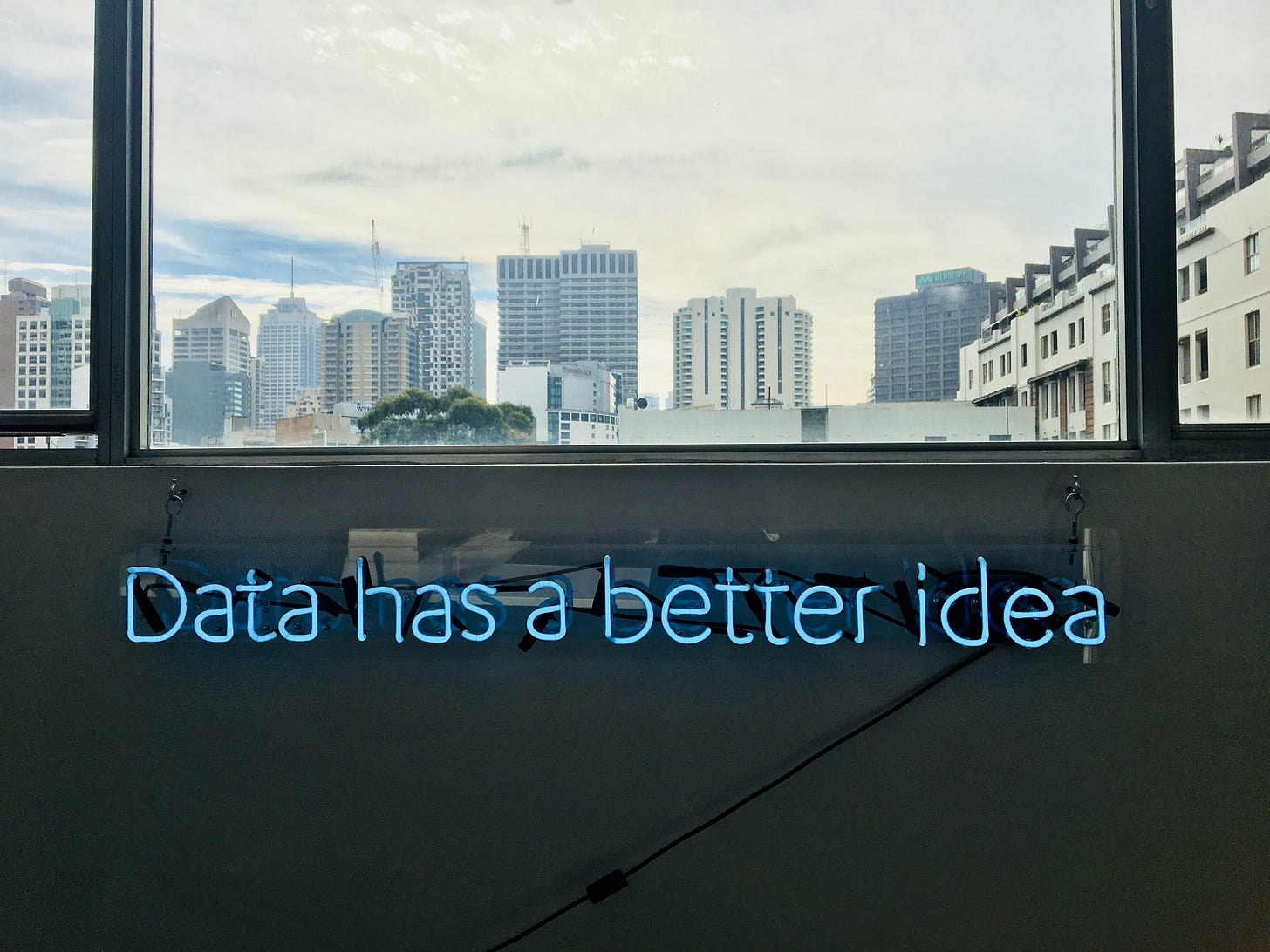
C./> HUMAN-CENTRIC DATA CENTER MANAGEMENT
Human beings will create a digital footprint, using machine-based devices, from the day they are born, untill the day they die, and sometimes beyond, when it commes to connections, memory, arts, commerce, rememberance,…”Cybernetic and Organic or ‘Cyborg Data’ will have to be considered
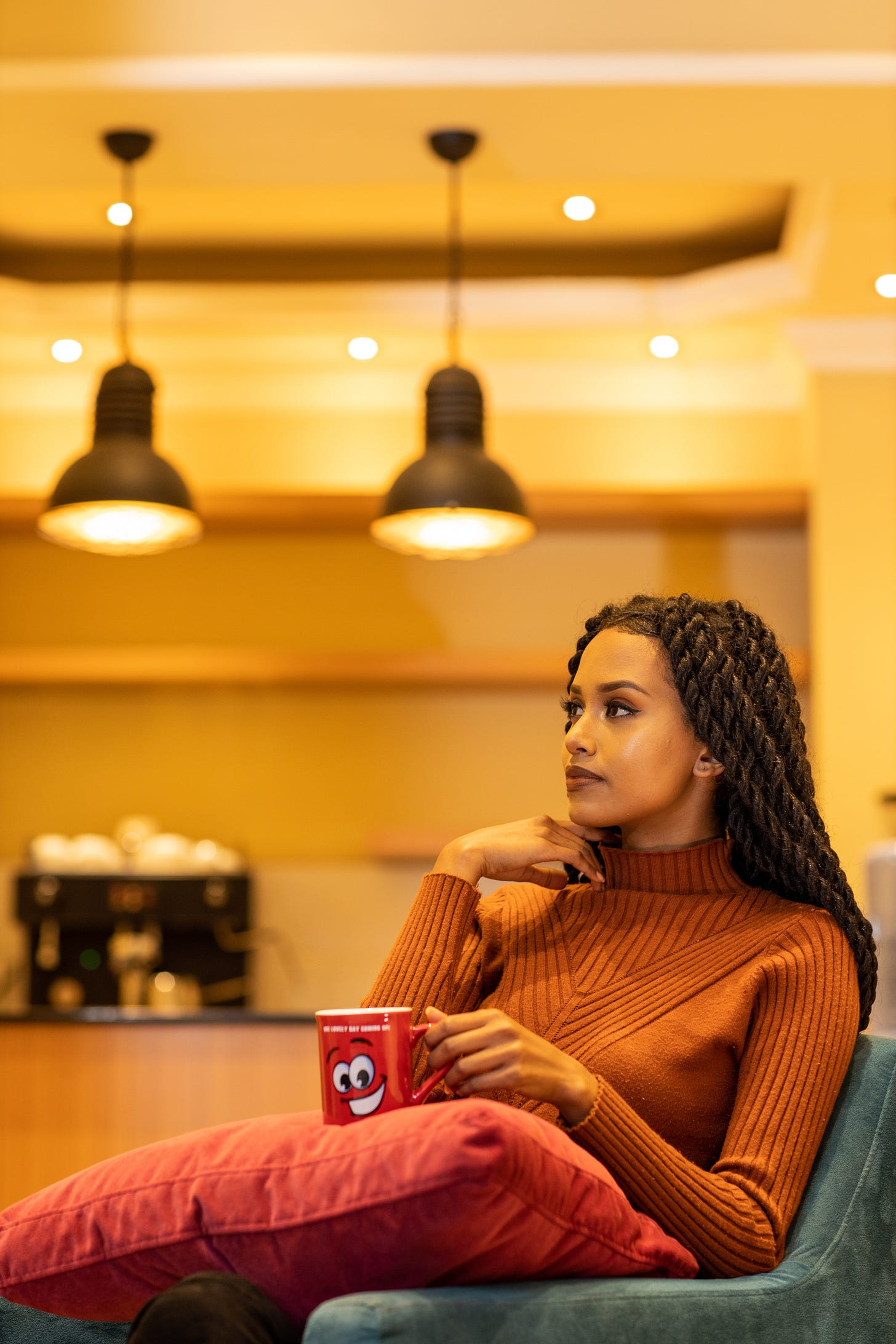
Following the core principle of using a Working software over ‘comprehensive documentation’….As a matter of facts too, machine will create a digital footprint that will be in use throughout its use and connections. “Machine Data will have to be considered”
Therefore… Managing a Data feed inside a data center, will mean observing ‘by default’, the following rule:
Human Data > Cyborg Data > Transactional data > Machine Data
With an agile warning system built upon the idea of Individuals and interactions over processes and tools, centered around pre-defined “Warning KPIs, or even Early Warining Indicators, EWIs’ setup in order to avoid breakdowns and malicious attacks.
AT GROUND or “Working” LEVEL, it means :
Social Media Data > Chatbot Data > Blockchain data > Disk space Data -
Blockchain technology will also provide cybersecurity oversight, quality (and auditing) control in database management, at least at transactional levels.
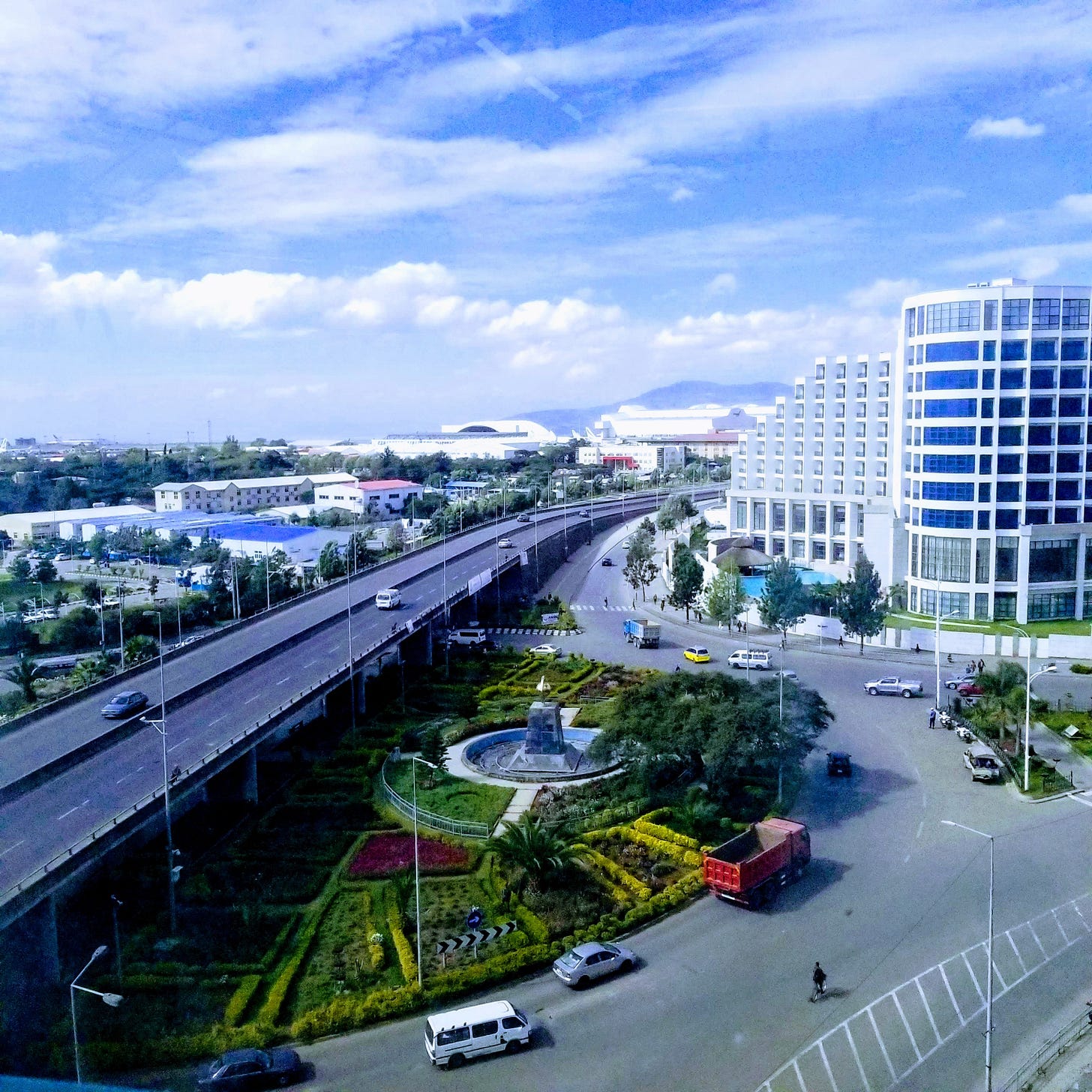
D./> NO EGO, ‘NO LOGO’ !
Managing a data center in the 21st century, will require a healthy approach to the idea of responding to change, over following a plan, and a total collaboration in “Ubuntu” fashion where all group of Data scientists, analysts, engineers, social media managers, …will collaborate fully with one another, and this, with NO or little EGO.
EGO = Being the first “Virus of the mind”, that tend to create siloes in industrial units. At a time when most Data teams are still exploring, and making new discoveries on a daily basis, having an ‘open-mid’ is KEY to good, functionning Data Mining/Exploring teams.
Whilst all Data teams, need to collaborate to extract the best value from Data, Data scientists, analysts, engineers, ..etc..
9. Ego could prevent full collaboration between team-members, we recommend ‘Agile Management’ and its many ‘derivatives’ as the best way to extract the most value out the data being prepared for analytics and decision-models.
Analytics are a necessity and should help build optimized decision-models, that should always be confirmed or RECONCILED at some point with the physical world ‘otherwise known as ‘PHYSICAL REALITY’ or, sometimes… ‘ORGANIC REALITY’
A.I = Artificial Intelligence is a necessity, but not the “Be all, and End All” either: A.I. should be SMART (Specific, Measurable, Achievable, Relevent and Time-bound).
A.I should be AUDITED on a regular basis (at least, Every 3 months) by Biological Human Beings.
A.I. should be broken down in clear stages and levels, in order to be QUALITY-CONTROLLED, by ‘Bio-Human beings’ with the right Data-science qualification
A.I should ALWAYS be QUALITY-CONTROLLED, on the ground too by ‘biological Human-beings”, in order to help MATCH IRL/IVL (In Real Life / In Virtual Life) with a constant ‘corrective feedback-loop’ with the peoples and systems, it is supposed to serve.
The 6 Elements of an AI Ecosystem:
Artificial intelligence ethics and governance models
Formal standards and procedures for the implementation of artificial intelligence engagements
Data and model management, governance and privacy
Understanding the human-machine integration, interactions, decision-support and outcome
Third-party AI vendor management
Cybersecurity vulnerability, risk management and business continuity
End.
#TEAMADMP.
Share this post